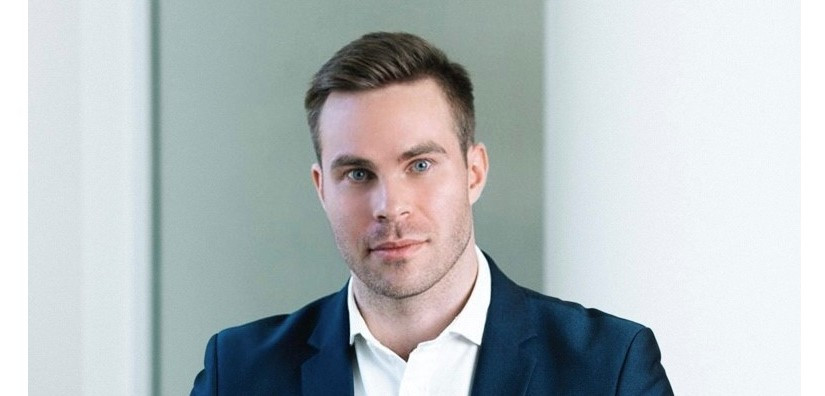
New era of risk management: role of machine learning and artificial intelligence
Traditionally, empirical research in finance strongly relied on statistical inference, but in recent decades rising computing power and data availability has resulted in many new opportunities for machine learning (ML) and artificial intelligence (AI). ML has become an important component in a range of financial services from asset and portfolio management, algorithmic trading, stress-testing, credit scoring to Regulatory Technology.
An increasing popularity and implementation of ML/AI in financial field, contribute to the fact that regulatory bodies have begun to issue discussion papers. Pioneering regulation paper has been issued in April 2011 by the Federal Reserve’s - Supervisory Guidance on Model Risk Management, and it was dedicated to assessing and management of model risk. Later in November 2019 Federation of European Risk Management Associations (FERMA) issued the paper on the implications of artificial intelligence (AI) for risk management. In April 2021, the European Commission published its proposed Regulation on Artificial Intelligence (draft Regulation), and in February 2022 The European Banking Authority (EBA) published a discussion paper on ML used in the context of internal ratings-based (IRB) models to calculate regulatory capital for credit risk.
What are ML models?
ML models is an expression of an algorithm that analyzes tons of data to find patterns or make predictions. Generally ML models falls into three categories:
- Supervised learning
- Unsupervised learning
- Semi-supervised learning.
Supervised learning is an approach to train ML/AI algorithm by using labelled data in order to classify new data or predict outcomes. In unsupervised learning you only have input data and model will find dependency without external interference. Semi-supervised learning is used with a very limited amount of data.
How can ML/AI help?
Traditionally, risk managers work with a limited number of drivers (15-25), implementation of an ML model can increase this amount to 99+. From a practical point of view, many of the ML/AI models are designed to achieve business needs, including, but not limited to, fraud detection, compliance, asset-liquidity management and credit scoring, stress-testing, capital calculation and many more. Big data and advanced analytics are opening new areas for more sophisticated models.
Just some brief examples where a management company can use ML/AI:
- Cluster analysis with machine learning: identify financial assets and divide them into groups that behave similarly in the markets in order to predict liquidity risks.
- Sentiment analysis and Natural Language Processing: collecting, identifying and analysing news regarding issuers and other financial market participants to predict changes in financial statements and compute credit risk using Altman Z-score model, or to monitor trader activity in order to detect and prevent illegal activity.
Implementation of ML/AI lies far beyond the examples I have provided. Just for volatility prediction a risk manager can use not only classic volatility prediction parametric methods like ARCH and GARCH, but also advanced data-driven methods like Support Vector Machines (SVM), Neural Network, and Deep Learning models. Of course, the difficulty increases exponentially when a management company uses more drivers in the models.
Limitations
- Data quality. Now more than ever is the definition “garbage in, garbage out” applicable to ML/AI models. Data that includes errors and outliers will significantly affect the final result from ML.
- Overfitting. When the model does not categorise the data correctly, because of overabundance of details and noise.
- Data access. There is regulation that limits the possibilities of collection and processing of big data repositories about “natural persons”.
- Black boxes. Many ML models are non-linear and very comprehensive.
- Skills. Appropriate skill is a crucial part of ML/AI implementation, otherwise there will be wasting time and resources.
Conclusion
The ML/AI algorithms can provide to risk manager opportunities in the way that traditional statistical approach could never hope to. Using an ML/AI model in risk management of day-to-day activity could significantly automate and optimise time to analyse risks and would help concentrate more on how to manage and mitigate them. Right now, we step into a new era – an era of ML and AI.
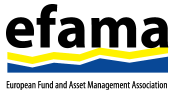
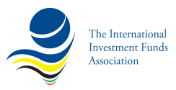
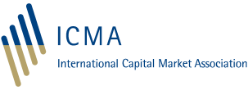